Track chairs:
Sana Ben Hamida – LAMSADE -Université Paris Dauphine – PSL (sana.mrabet@dauphine.psl.eu)
Amel Borgi – LIPAH Université Tunis El Manar (amel.borgi@insat.rnu.tn)
Track Description:
Automatic decision in Intelligent Systems is based essentially on smart data exploitation and analysis. Machine learning (ML) provides in this context a mechanism to process and analyse large amounts of data and make more informed decision based on the resulting analysis. However, due to the increased need for understanding and analysing the large amount of data, traditional ML algorithms need to be extended with additional paradigms to boost their efficiency in providing data driven predictions and decisions. Learning paradigms help to better gain insights the behaviour of the training data and offer some solutions to deal with big data challenges such as volume, velocity and variety. For example, Active Learning approaches improve ML algorithms’ ability to better process voluminous or/and imbalanced data; Ensemble learning combines multiple learners to obtain better learning outcomes and decisions; Online Learning offers advanced tools to learn from streaming big data; Incremental learning is applied to alleviate challenges of data availability, Federated learning enables multiple actors to collaboratively learn a ML model without sharing data and thus without compromising data security, Explainable AI provides tools and frameworks to help us understand and interpret predictions made by ML models, etc. This conference track is looking for emerging research in machine Learning paradigms applied to either address big data challenges or to improve predictions and decision making for intelligent systems. Submitted papers must describe original research in the following (but not limited) topics:
- Active Learning to address big/imbalanced data challenges
- Ensemble Learning to improve decision making
- Learning Paradigms for recommender systems
- Transfer Learning in the context of incomplete data
- Online (Transfer) Learning for streaming big data
- Federated learning to exploit decentralised data securely
- Explainable Artificial Intelligence for machine learning
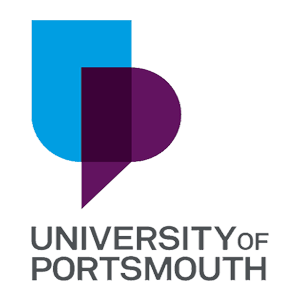
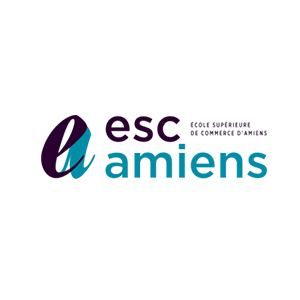
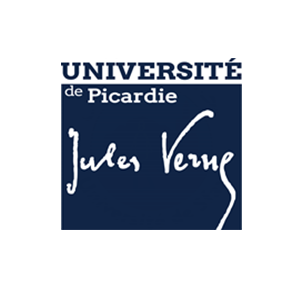
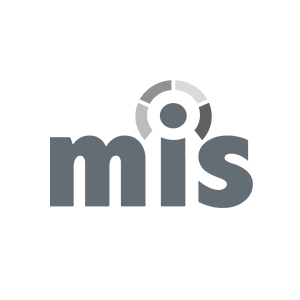
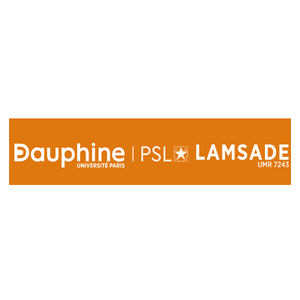
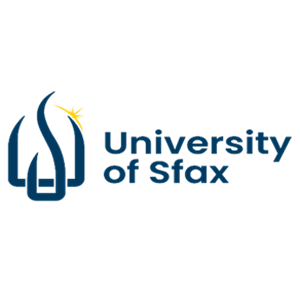
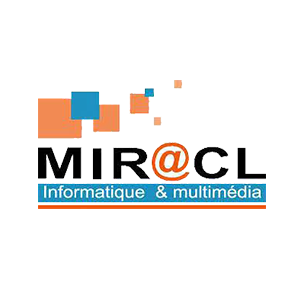
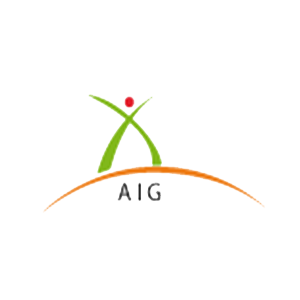